For Japanese
Biography
Profile
- Name: Satoshi Tanaka
- Twitter: @scepter914
- Github: @scepter914
Work Experience
- Apr. 2020 - Now, TIER IV, Inc. Autonomous Driving Sensing/Perception Engineer
- Internship
- Apr. 2018 - Apr. 2019, Internship at Preferred Networks, Inc. as a part-time engineer
- Aug. 2017 - Mar. 2018, Internship at Hitachi, Ltd as a research assistant
Academic Background
- Master’s Degree in Information Science and Engineering, the University of Tokyo
- Apr. 2018 - Mar. 2020, Ishikawa Senoo Lab, Department of Creative Informatics, Graduate School of Information Science and Technology
- Bachelor’s Degree in Precision Engineering, the University of Tokyo
- Apr. 2017 - Mar. 2018, Kotani Lab, Research Center for Advanced Sceience and Technology
- Apr. 2016 - Mar. 2018, Dept. of Precison Engineering
- Apr. 2014 - Mar. 2016, Faculty of Liberal Arts
Interest
- Robotics, Computer Vision, Control theory
- High-speed Robotics
- System integration of high-speed robot using 1000fps high-speed image processing
- Deformation Control, robot force control for dynamic manipulation with high speediness
- Application of high-speed visual control for logistics, Unmanned Aerial Vehicle(UAV)
- Robot vision
- 3D perception for robotics with sensor fusion
- Other hobby
Publication
International Conference (First author)
- Satoshi Tanaka, Keisuke Koyama, Taku Senoo, Makoto Shimojo, and Masatoshi Ishikawa: High-speed Hitting Grasping with Magripper, a Highly Backdrivable Gripper using Magnetic Gear and Plastic Deformation Control, 2020 IEEE/RSJ International Conference on Intelligent Robots and Systems (IROS2020), Proceedings, pp. 9137 - 9143. [2020 IEEE Robotics and Automation Society Japan Joint Chapter Young Award]
- Satoshi Tanaka, Keisuke Koyama, Taku Senoo, and Masatoshi Ishikawa: Adaptive Visual Shock Absorber with Visual-based Maxwell Model Using Magnetic Gear, The 2020 International Conference on Robotics and Automation (ICRA2020), Proceedings, pp. 6163-6168.
- Satoshi Tanaka, Taku Senoo, and Masatoshi Ishikawa: Non-Stop Handover of Parcel to Airborne UAV Based on High-Speed Visual Object Tracking, 2019 19th International Conference on Advanced Robotics (ICAR2019), Proceedings, pp. 414-419.
- Satoshi Tanaka, Taku Senoo, and Masatoshi Ishikawa: High-speed UAV Delivery System with Non-Stop Parcel Handover Using High-speed Visual Control, 2019 IEEE Intelligent Transportation Systems Conference (ITSC19), Proceedings, pp. 4449-4455.
International Conference (Not first author)
- Taisei Fujimoto, Satoshi Tanaka, and Shinpei Kato: LaneFusion: 3D Object Detection with Rasterized Lane Map, the 2022 33rd IEEE Intelligent Vehicles Symposium (IV 2022), Proceedings, pp. 396-403.
Other publication
- Kazunari Kawabata, Manato Hirabayashi, David Wong, Satoshi Tanaka, Akihito Ohsato AD perception and applications using automotive HDR cameras, the 4th Autoware workshop at the 2022 33rd IEEE Intelligent Vehicles Symposium (IV 2022)
Award, Scholarship
- 2020 IEEE Robotics and Automation Society Japan Joint Chapter Young Award
- 2019/04-2020/03 (1 year) Toyota Dowango scholarship for advanced artifical intelligence researcher
- 2018/04-2019/03 (1 year) Toyota Dowango scholarship for advanced artifical intelligence researcher
Projects
mmCarrot
DepthAnything-ROS
(Research) LaneFusion: 3d detection with HD map
- Accepted at IV2022
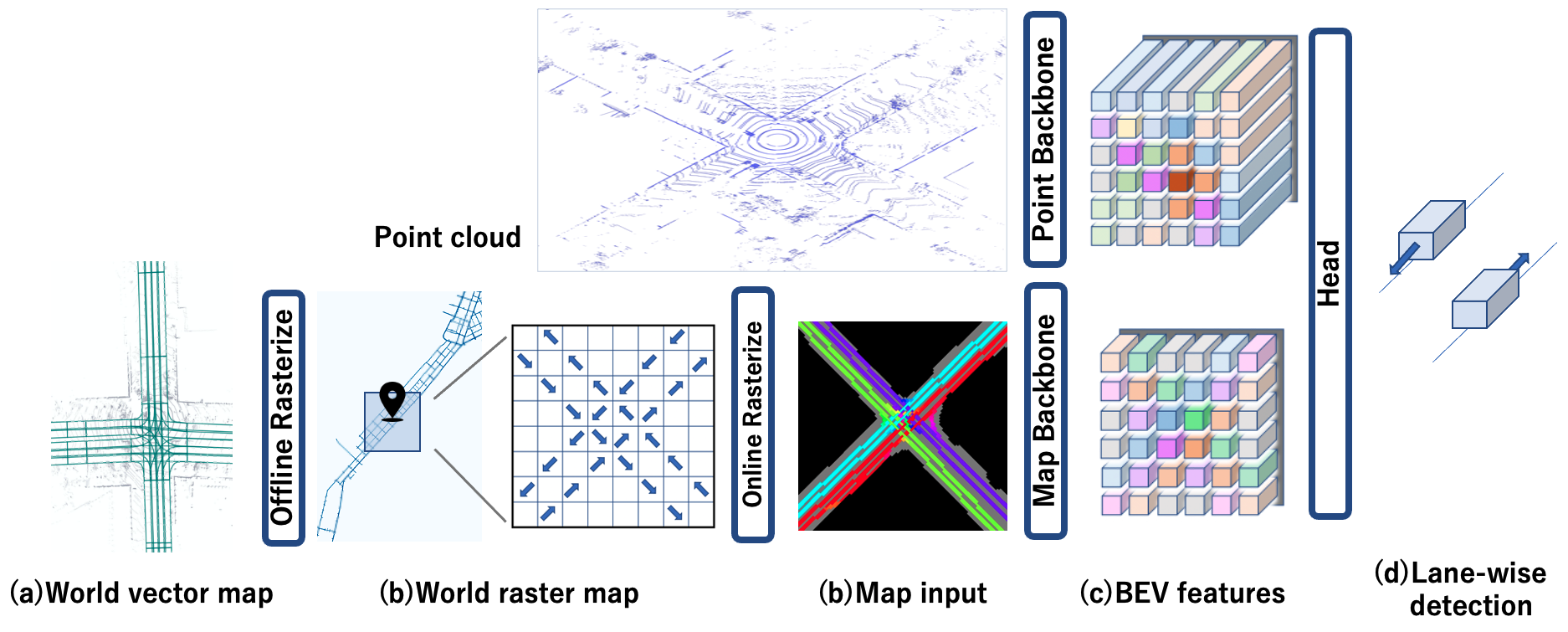
(Research) High-speed Hitting Grasping with Magripper
- Accepted at IROS2020 [2020 IEEE Robotics and Automation Society Japan Joint Chapter Young Award]
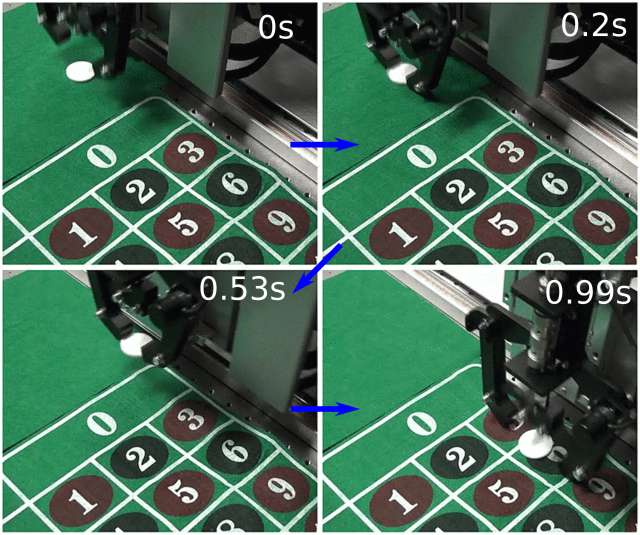
(Research) Adaptive Visual Shock Absorber with Magslider
- Accepted at ICRA2020
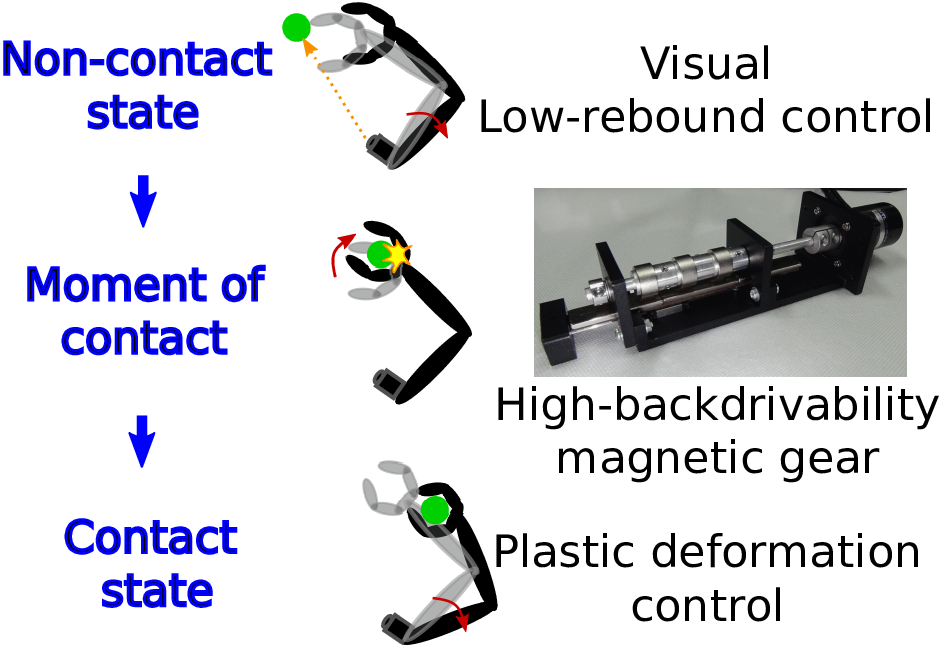
(Research) High-speed supply station for UAV delivery system
- Accepted at ITSC2019
Robotic Competition
- Team Leader for ABU Robocon2016
- Winner of National Championships, 2nd-runnerup of ABU Robocon, ABU Robocon award.
- Visited to the prime minister’s residence as the team leader of representation from Japan team. Reported by link and link.
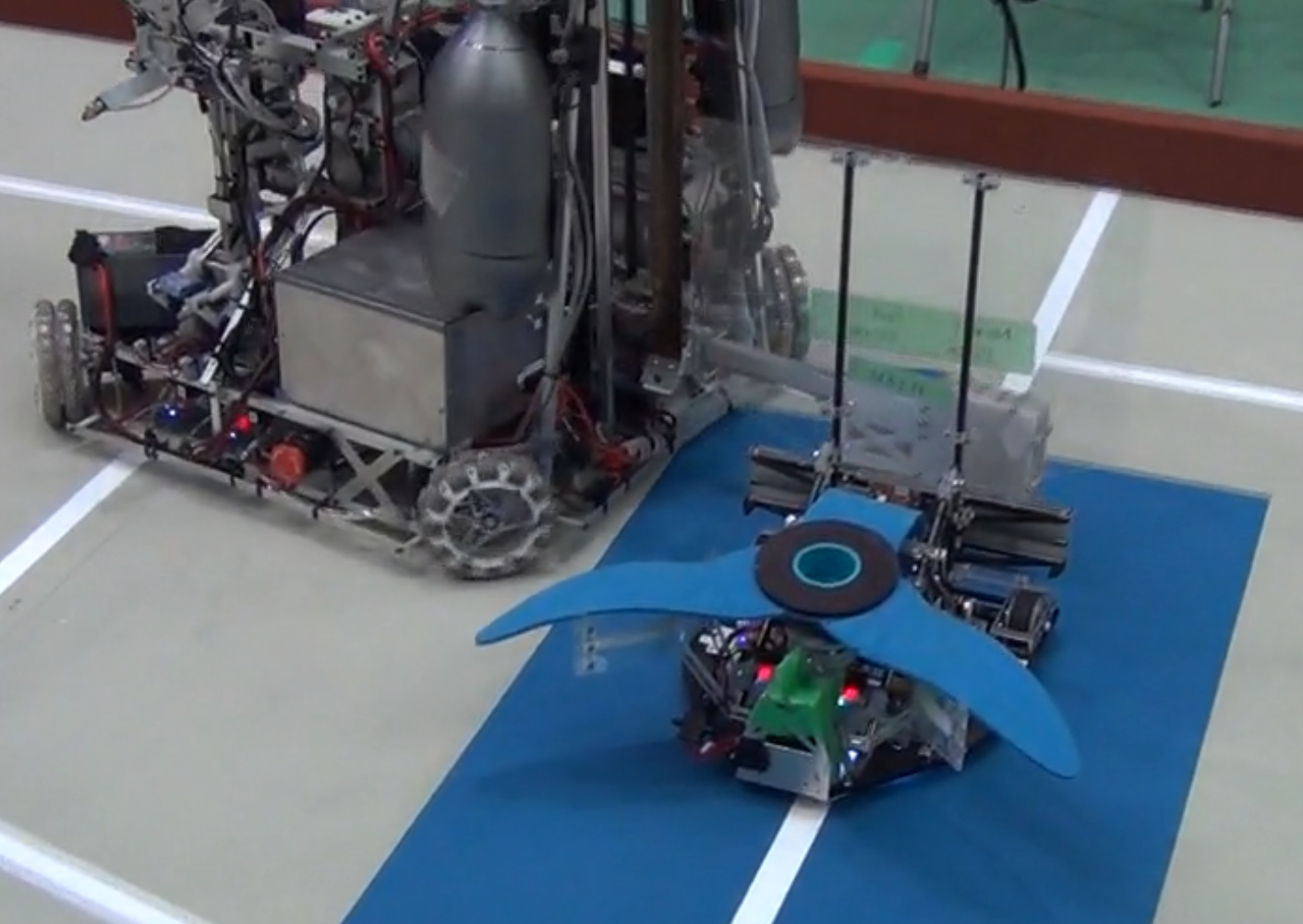
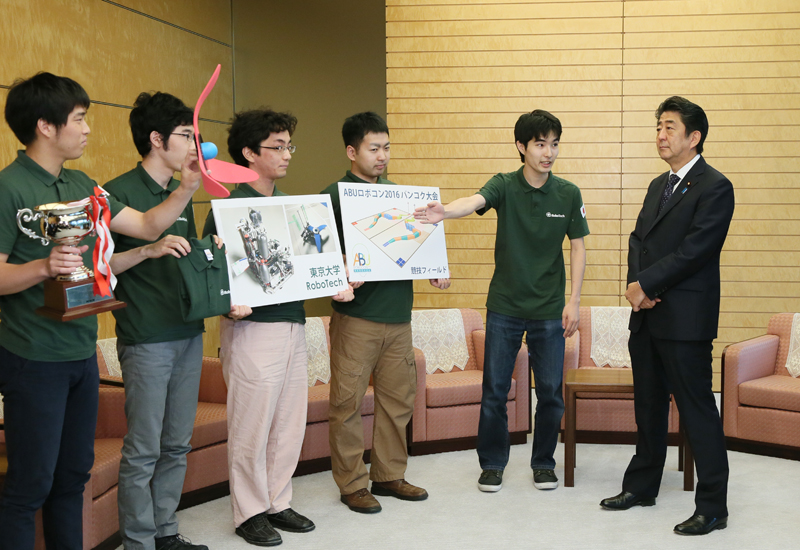
Other projects
- Mouse for CAD
- Maker Faire Tokyo 2017, GUGEN2017
- Youtube link